Marketing and Sales Funnel Math
Estimating digital marketing campaign performance under uncertainty
In a previous blog post we looked at the question
How much traffic do you need in your marketing and sales funnel and what does it cost?
We built a basic spreadsheet tool for planning our digital marketing campaign. It gives us
- A way to estimate campaign performance
- An outlook for our contribution margin
- Reality checks for feasibility
- A tool to compare alternative campaigns and channels.
Don’t worry if you did not get all the steps right or if you simply don’t have the time for it. You can download the template from the idea,- cast briefing center (log-in required).
Now we are taking it a bit further. The question we will explore is
How to estimate digital marketing campaign performance under uncertainty?
We will build on the same example case study of selling a BBQ grill set. And we will use the data we obtained from the Google Keyword Planner to apply some further concepts and tools.
What do we mean by uncertainty and VUCA in the context of digital marketing?
The acronym VUCA stands for Volatility/Vulnerability, Uncertainty, Complexity and Ambiguity. In the context of the digital marketing campaign and the marketing and sales funnel we encounter these challenges in different areas. Let’s see how this applies to our BBQ grill product in our case study:
Volatility and Vulnerability
The product supply chain for our BBQ grill set may be vulnerable. A dramatic change in weather conditions may cause the shipping container to disappear or arrive too late. There is little to gain from a bunch of BBQ grills which arrive during snow season.
Uncertainty
We do not know what our conversion rate for the campaign and the product is. It may be as low as 0% and as high as 100%. Though a realistic estimate might in the range of 2% – 10% with a most likely value of 5%.
Complexity
The word-of-mouth may create a non-linear effect on product adoption. This could work both ways: if the product is great then sales goes through the roof and we may run out of stock quickly. If the product has a quality issue the word-of-mouth works against us and an avalanche of product returns could hit us. The recalled and returned items pile up to the roof and staff is busy is fixing the mess.
Ambiguity
When you are planning to sell a new product into a new market you are facing a lot of ambiguity. In other words, you do not know much about the situation and you have no previous experience and evidence. For example, if you are planning to sell a solar powered BBQ grill set to customers in Iceland (No offence!) you really don’t know if it words at all if anybody would buy it. Huh!
Dealing with uncertainty in digital marketing campaigns
Coming back to the data of our BBQ grill set case study, we first need to identify areas where uncertainty applies. In the case of our KPIs (Key Performance Indicators) for the digital marketing campaign we can identify at least three areas:
- CPC, Cost-per-Click
- CTR, Click-through-rate
- Conversion rate
Note: the term “rate” is technically not correct. A rate refers to an amount or volume over time, for example unit sales per month. In system dynamics we call this a flow. In contrast a “conversion rate” is actually the fraction of customers purchasing a product.
Let’s see how you can articulate uncertainty and build this into the spreadsheet model for estimation of traffic and budget requirements.
We do want to get an answer to the questions:
- Reach: What is the range of traffic to our website we should expect from this campaign?
- Budget: What is a realistic assumption for the budget requirement?
- Profit contribution: What is the probability that this campaign will generate a positive return or a loss?
You should get an answer for your own planning and for your peace of mind. You may want to bet on the winning campaign. And if you are reporting to a manager, you should be able to defend your budget request with data and support an informed decision.
How to estimate parameters for the campaign performance?
First, let’s go back to our sample data which we obtained from the Google Keyword Planner. We can see that both CPC and CTR show a variability.
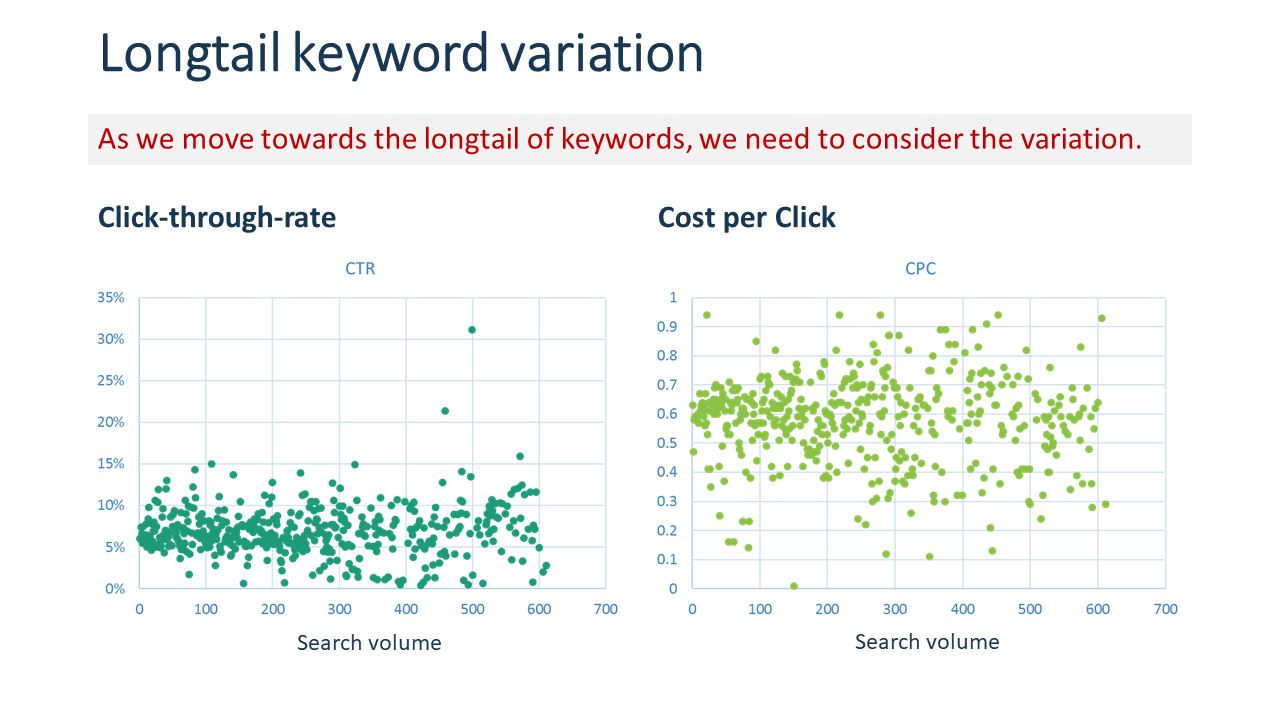
Moving towards the long-tail of keywords shows increased variation in cost and conversion. VUCA
We can use these data to estimate a distribution which tells us the probability for this this parameter. The distribution of CTR shows some density around 7%. But it can be as low as close to 0% and in rare cases as high as 30%.
Estimating probability of CTR
After fitting a statistical distribution to the data, we get the parameters for our estimated probability distribution with a mean of 6.9% and a standard deviation of 0.03. This enables us to generate a sample by making random draws from this distribution.
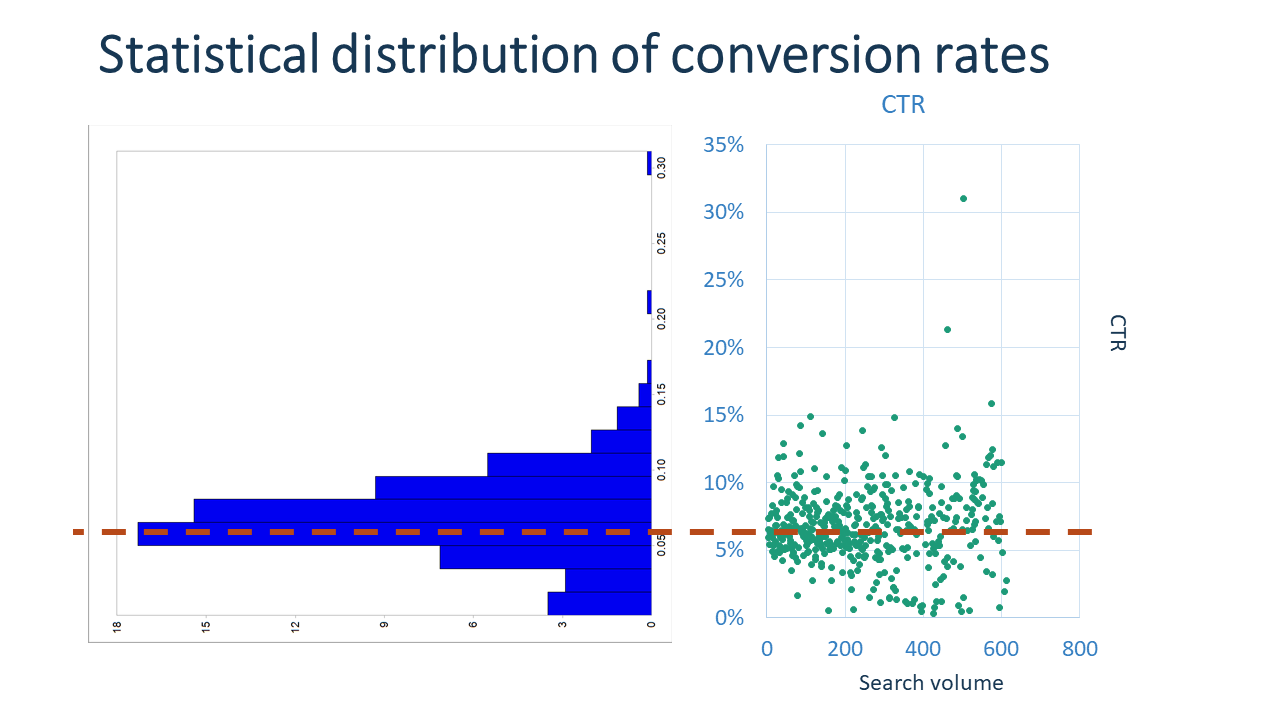
Search volume and CTR in a scatter plot. We can estimate the expected value of CTR and conversion rate. This sample is based on keyword research report from Google Search Analytics or Bing Sarch Analytics.
Estimating CPC
We can do the same for estimating CPC. Again, we take our sample data and fit a distribution to the data. Here you can see that this is not a random normal distribution, technically speaking. We need to be careful in selecting the right distribution we want to fit to the data sample. Otherwise we will get distorted estimates later on.
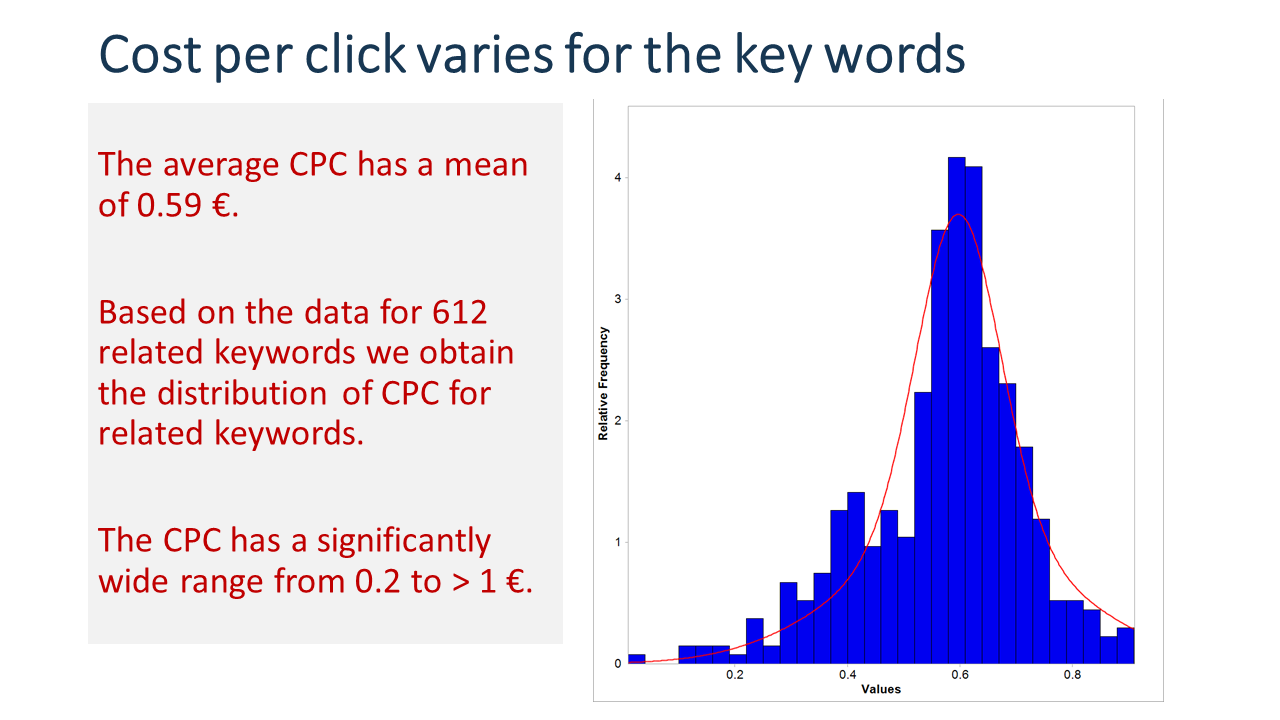
Based on a data sample we can estimate the distribution of cost per click. This can be used as an input parameter for the spreadsheet model to estimate web traffic and marketing budget requirements.
Estimating the Conversion Rate
As a last step we need to estimate the conversion rate. This is a little bit more tricky since there are several possibilities how to get estimates:
- Either we have data from comparable test campaigns,
- Or we can rely on insights from previous campaigns.
- In our example we do not have any previous insights. Therefore, we need to create an expert estimate.
We can also articulate this in form of a probability distribution. What we need is three anchor points for what we believe the conversion rate would be:
- The minimum value. Let’s we assume that at least we should be able to generation 0.5% conversions.
- The mode: this is the most likely value. Let’s say 5% conversion.
- The maximum value. We assume that it is unlikely that conversion exceeds 7%.
Including expert judgement is an elegant way to include assumptions about uncertainty in your project plan. It also helps to make the implicit assumptions transparent and foster a dialogue about your “mental model” of situation.
This will give us a distribution which looks like this, from which we can draw random samples for our estimation.
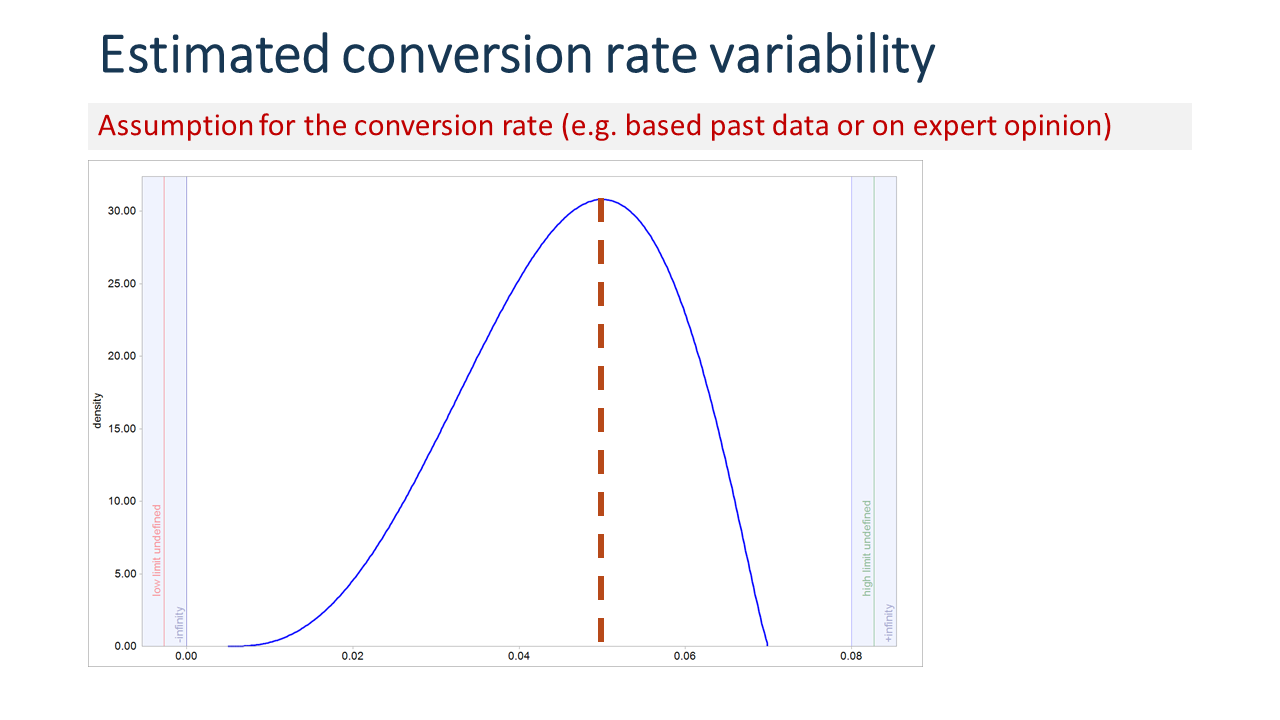
How to define the parameters for conversion rate under uncertainty? Uncertainty means that the true parameter is not yet known and / or shows variability. The conversion rate can be expressed by a PERT or Triangular distribution.
Simulation and estimation of budget requirement under uncertainty
Now we are ready to plug this into our spreadsheet. Instead of a fixed value for CTR, CPC and Conversion Rate, we are now taking random samples for each one of these parameters. We will do this for a large number of calculations, let’s say 1’000. This approach is called Monte-Carlo-Simulation.
If you are not familiar with this approach or if you are not there yet to use and present the numbers with confidence, you can take a minimalistic approach. At a minimum you can use the built-in scenario functionality of your spreadsheet software and create different scenarios for minimum, maximum and most likely setting. This is a first step, though it does not give you all the answers we are looking for.
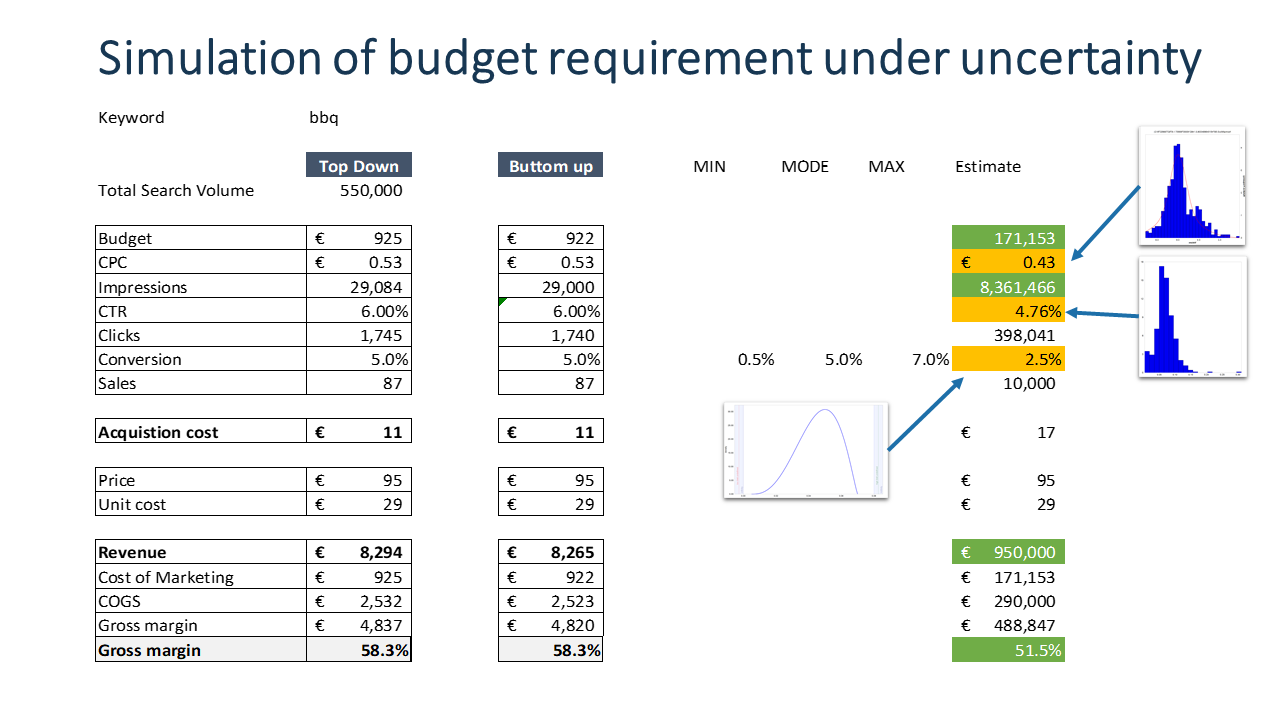
Spreadsheet for estimating top down and bottom up: Budget required, Campaign performance, Campaign cost, CPC, CTR, Clicks, Conversion rate.
Simulation results
Let’s take a look at the simulation results. Keep in mind that we want to generate 10k unit sales.
Estimated required search volume under uncertainty
First, the distribution of the required search volume we should get from our SEM, Search Engine Marketing, looks like this. We should get at least 150k clicks and visits to our website or landing page. Assuming 200k is a safer bet. Also, it is fair to assume that the campaign would generate well above 150k visitors.
You can also use this value for a check-point review or for setting targets for the team and for external marketing partners and vendors.
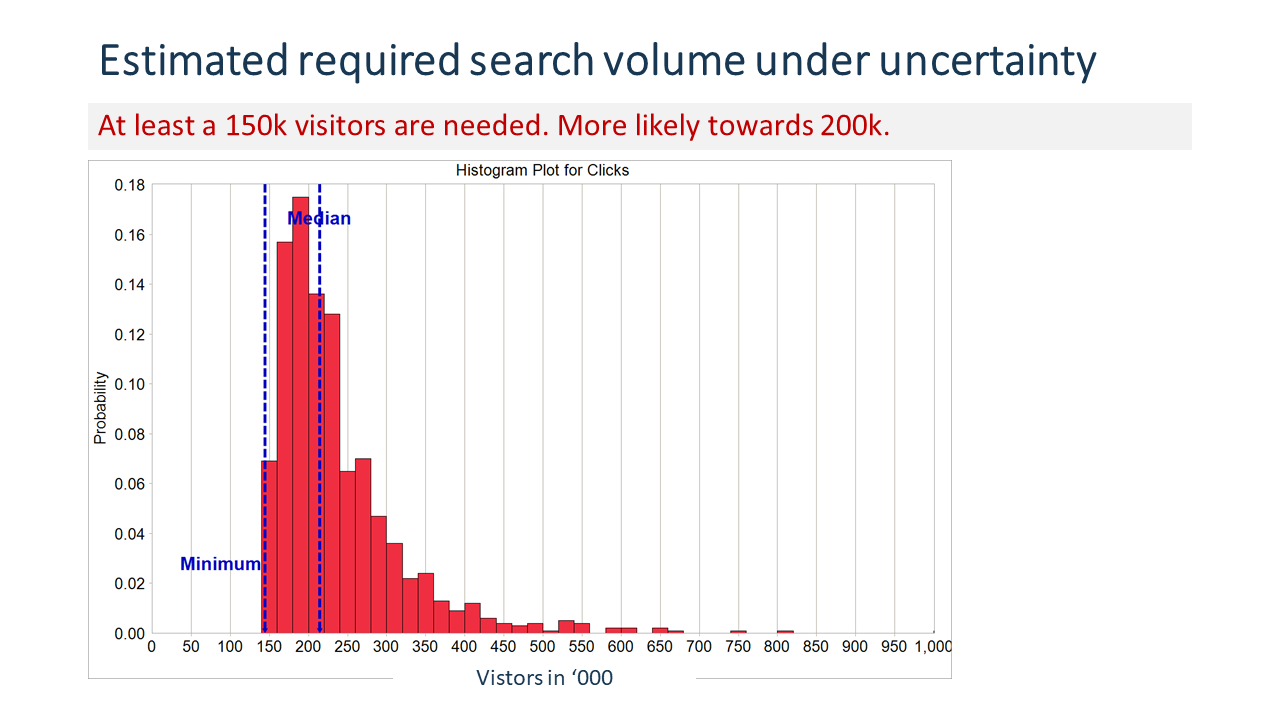
Estimated search volume. Statistical distribution under uncertainty.
Estimated budget under uncertainty
In terms of budget we should assume that about 140 will be required. This is the most likely value. With a probability of 80% the budget will fall into the range of 80k – 210k. That is quite a wide range of course. We should therefore seek ways to reduce the variation. The first step would be to ask what is driving this variation?
Estimation under uncertainty helps to make better informed decisions.
Why? Compare the 140k to the 106k we estimated with fixed assumptions. That is quite a difference. If you would ask for 106k budget, chances are that it will not be sufficient, and you will have to ask for more.
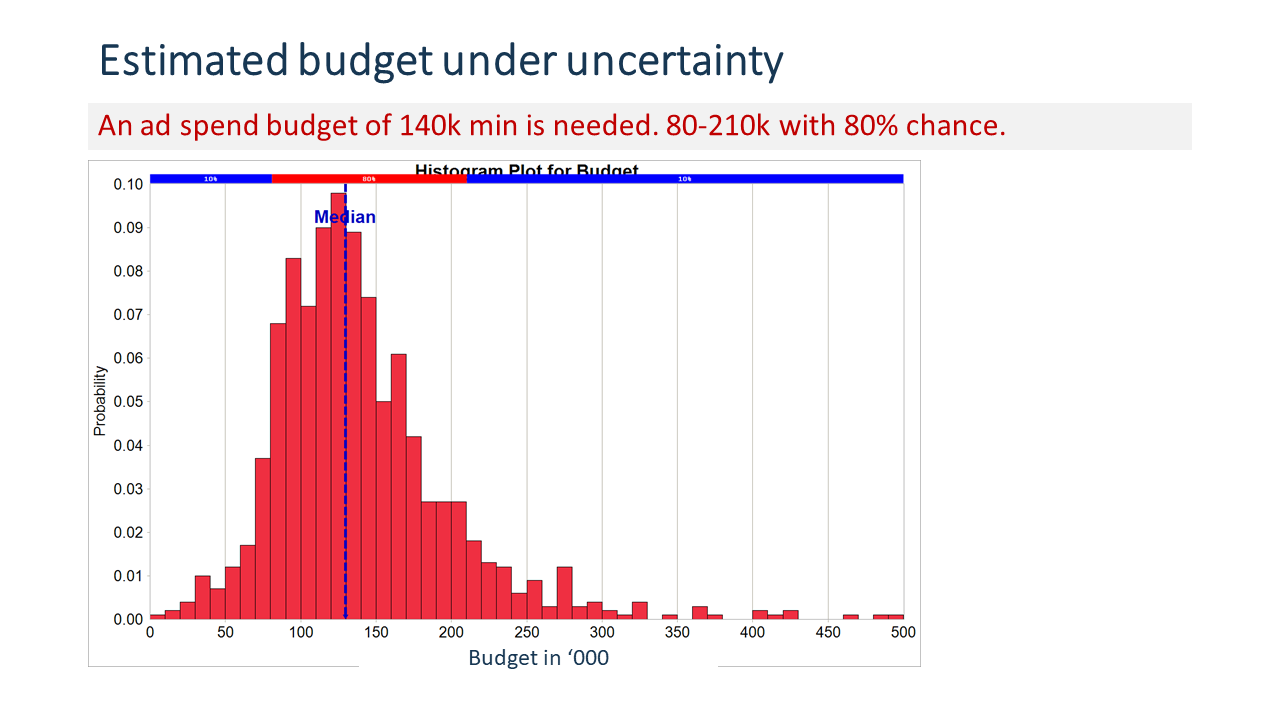
Statistical distribution of estimated budget under uncertainty.
Estimated impact on profit under uncertainty
The last question is maybe the most important one:
What are the chances that this campaign will be profitable or generate a loss?
There is only a slim chance that the campaign will generate a loss. (<0.01% probability). The expected margin will be around 54%. And there is even some upside. The probability that the gross margin will be higher than 54% is 36%.
You could also argue of course that there is 64% chance that the margin is below 54%. This information is highly relevant if you compare this campaign to another alternative which would generate a similar return. Then you want to pick the one with the highest upside opportunity and lowest downside risk.
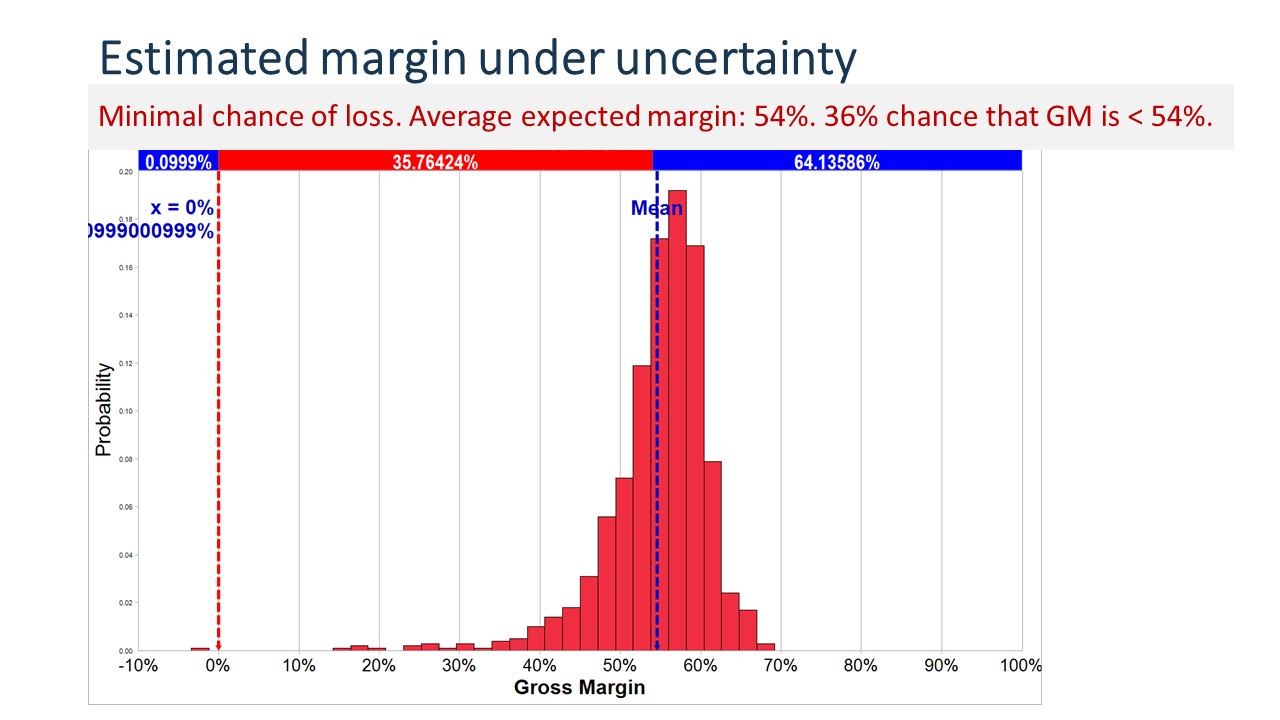
Statistical distribution of estimated margin under uncertainty.
Seasonality impact on campaign success
In our BBQ grill set example we also need to take seasonality effects into consideration. The best chance to sell a BBQ grill set in our target geography is between March and September. At a minimum, take a look at the search volume over time. This will give you some indication if there is a seasonality and what it means for the timing of the campaign.
You can also take a look at the change in CPC over time, because popular and high performing keywords become usually more expensive during high season.
You can get all these data points and insights from the data which are made available by the search engine providers. We are not covering how to factor in in seasonality into your estimation. I leave this to you for now or to a topic in the group coaching program.
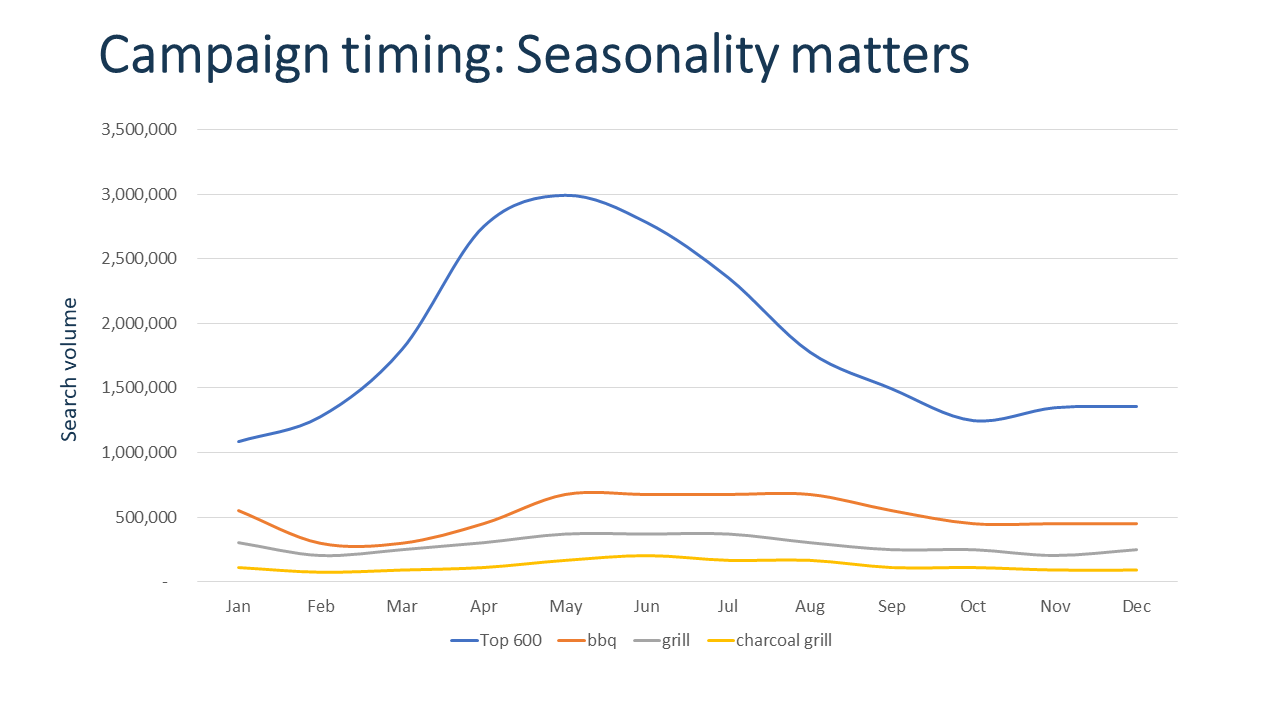
Source: Google Keyword Planner. Timing campaigns is important. The addressable market changes in industries with seasonalities. This image shows the trends in the outdoor equipment (BBQ) market.
Conclusion
We looked at an example case study of selling BBQ grill sets through a digital marketing and sales campaign. We started out by putting a basic model together. In this blog post we took it a step further and added uncertainty to our plan.
- This helps us to answer key questions such as:
- What is the range of traffic volume we should expect?
- What is the range of the required budget?
- What is the likelihood that this campaign generates a profit or loss?
We accomplished this by including assumptions for variability of the KPI parameters CPC, CTR and Conversion Rate into our spreadsheet model. This gives us a new dimension of looking at the campaign performance. And it helps to make better informed decisions and selecting winning projects and campaigns.
Guidance for marketing executives and decision makers:
Ask your team and vendors to give you the assumptions or a complete sensitivity analysis.
The higher the impact, the more budget, the better your estimation and the more effective and proactive your de-risking approach should be.
Related Material
Check out the WITTIGONIA YouTube Channel and the idea,- podcast series.
TL;DR
Reverse calculate how much budget you need for your campaign. Apply sensitivity analysis to deal with uncertainty of the key parameters. Kick back and watch the video.